5 Algorithms that Demonstrate Artificial Intelligence BiasIntroductionThe unfortunate truth about our society is that bias is ingrained in human nature. Humans may be biased intentionally against members of certain racial or religious minorities, genders, or nationalities, or they may be biased unintentionally due to social conditioning from birth, family history, and society. For whatever reason, people are biased, and these biases are now passed on to artificial intelligence systems that are developed by humans. 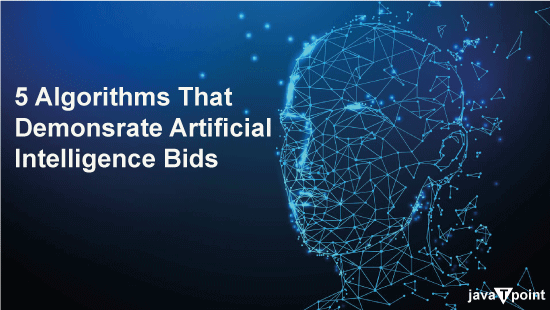 Artificial intelligence systems that are trained on data containing human biases, historical inequalities, or various metrics of judgment based on human gender, race, nationality, sexual orientation, etc., may inherit these biases from humans into Artificial Intelligence Bias. For instance, Amazon discovered that their AI recruiting algorithm had a bias against women. The number of resumes submitted and the number of hired candidates over the previous ten years served as the basis for this algorithm. Since, the majority of the candidates were men, the algorithm favored men over women. This example demonstrates how biases in artificial intelligence can have a significant negative impact. This bias undermines the biased group's ability to fully participate in the world and contribute equally to the economy. And, while it harms the groups against which the algorithm is biased, it also harms humans' trust in artificial intelligence algorithms to function impartially. It reduces the chances that artificial intelligence will be applied to all facets of business and industry since it fosters mistrust and the worry that individuals might face discrimination. Therefore, before releasing these artificial intelligence algorithms onto the market, the technical industries that create them have to ensure that they are free of bias. Businesses can accomplish this by promoting AI bias research in an effort to end bias in the future. However, before this can happen, we must first understand the examples of Artificial Intelligence Bias as demonstrated by various algorithms. So, let's examine them in order to comprehend what algorithms should not perform in the future. What is AI biasArtificial intelligence (AI) bias, also known as machine learning bias or algorithm bias, is the phenomenon of biased outcomes resulting from human biases that distort the original training data or AI algorithm, potentially producing harmful outcomes. Unaddressed AI bias can have a negative effect on an organization's performance as well as people's capacity to engage with the economy and society. Bias reduces AI accuracy, and thus its potential. Systems that yield skewed results are less likely to be profitable for businesses. Furthermore, mistrust among women, people of color, people with disabilities, the LGBTQ community, and other marginalized groups may be fostered by scandals resulting from AI bias. AI models absorb societal biases that can be quietly embedded in the mountains of data they are trained on. In use cases like hiring, policing, credit scoring, and many more, historically marginalized groups may suffer harm as a result of historically biased data collection that reflects societal inequity. Which algorithms demonstrate Artificial Intelligence Bias?Below are a few algorithms that have shown Artificial Intelligence Bias. Notably, this bias is always demonstrated against minority groups, such as black people, Asian people, women, and so on. - COMPAS Algorithm biased against black people
COMPAS, which stands for Correctional Offender Management Profiling for Alternative Sanctions, is an artificial intelligence algorithm developed by Northpointe that is used in the United States to predict which criminals are more likely to reoffend in the future. Judges make decisions about these criminals' futures based on these forecasts, including their jail sentences and bail amounts for release. ProPublica, a nonprofit news organization that has won the Pulitzer Prize, discovered that COMPAS was biased. Black criminals were found to be far more likely to commit crimes again in the future than they were to commit them in the first place. White criminals, on the other hand, were judged to be less risky comparatively. Black criminals were mistakenly classified as more dangerous nearly twice as often as white criminals, even in cases of violent crimes. This discovery in COMPAS demonstrated that it had somehow learned the common human bias, which is that black people commit far more crimes than white people on average and are more likely to commit crimes in the future as well. - PredPol Algorithm biased against minorities
PredPol, also known as predictive policing, is an artificial intelligence algorithm that aims to predict where crimes will occur in the future using crime data collected by police, such as arrest counts, the number of police calls in a given area, and so on. The goal of this algorithm, which is already in use by US police departments in California, Florida, Maryland, and other states, is to lessen human bias in the department by entrusting artificial intelligence with crime prediction. However, researchers in the United States discovered that PredPol was biased, sending police officers to neighborhoods with a large number of racial minorities regardless of crime rates. This was due to a feedback loop in PredPol, in which the algorithm predicted more crimes in areas where more police reports were filed. However, it's possible that a higher concentration of police-possibly as a result of existing human bias-led to a higher number of police reports in these areas. Additionally, this led to a bias in the algorithm, which caused more police to be sent to these areas. - Amazon's Recruiting Engine biased against women
The Amazon recruiting engine is an artificial intelligence algorithm designed to analyze the resumes of job applicants applying to Amazon and determine which ones will be contacted for further interviews and selection. Amazon created this algorithm to automate their search for talented individuals and eliminate the inherent human bias that exists in all human recruiters. However, the Amazon algorithm proved to be biased against women during the recruitment process. This could have happened because the recruiting algorithm was trained to analyze candidates' resumes by studying Amazon's responses to resumes submitted in the previous ten years. However, the human recruiters who previously analyzed these resumes were mostly men, with an inherent bias against female candidates, which was passed on to the AI algorithm. When Amazon studied the algorithm, they discovered that it automatically handicapped resumes containing words like "women" and downgraded graduates of two all-women colleges. As a result, Amazon discarded the algorithm and stopped using it to evaluate candidates for recruitment. - Google Photos Algorithm biased against black people
Google Photos includes a labeling feature that adds a label to a photo based on what is shown in the picture. This is accomplished by a Convolutional Neural Network (CNN), which employs image recognition to tag the photos after being trained on millions of images through supervised learning. When a black software developer and his friend's photos were classified as gorillas by Google, the algorithm was found to be racist. Google stated that they were appalled and sincerely sorry for the error, and promised to correct it in the future. However, until two years later, Google had only removed gorillas and other types of monkeys from the Convolutional Neural Network vocabulary, ensuring that no photo was identified as such. Google Photos returned "no results" for all monkey-related search terms, including gorilla, chimp, and chimpanzee. However, this is only a temporary fix because it does not address the underlying issue. Image labeling technology remains imperfect, and even the most complex algorithms rely solely on their training, with no way to detect corner cases in real life. - IDEMIA'S Facial Recognition Algorithm biased against black women
IDEMIA's is a company that develops facial recognition algorithms for use by police forces in the United States, Australia, France, etc. In the USA, this facial recognition system analyzes about 30 million mugshots to determine whether or not an individual is a criminal or a threat to society. When compared to white women or even both black and white men, the National Institute of Standards and Technology discovered that the algorithm significantly misidentified black women. According to the National Institute of Standards and Technology, Idemia's algorithms incorrectly matched a white woman's face at a rate of one in 10,000 and a black woman's face at a rate of one in 1,000. This is 10 times more false matches for black women, which is a lot! Facial recognition algorithms are generally considered acceptable if their false match rate is one in 10,000, but the false match rate for black women was significantly higher. Idemia claims that the NIST-tested algorithms have not been commercially released and that their algorithms are improving at different rates as physical differences between races emerge.
|